SOCR EduMaterials FunctorActivities Bernoulli Distributions
From Socr
(Difference between revisions)
Line 16: | Line 16: | ||
* '''Exercise 2:''' Use SOCR to graph and print the MGF of the distribution of a geometric random variable with <math> p=0.2, p=0.7 </math>. What is the shape of this function? What happens when <math> p </math> is large? What happens when <math> p </math> is small? | * '''Exercise 2:''' Use SOCR to graph and print the MGF of the distribution of a geometric random variable with <math> p=0.2, p=0.7 </math>. What is the shape of this function? What happens when <math> p </math> is large? What happens when <math> p </math> is small? | ||
- | * '''Exercise 3:''' You learned in class about the properties of MGF's If <math> X_1, ...X_n are iid. and Y = \sum_{i=1}^n X_i. </math> then | + | * '''Exercise 3:''' You learned in class about the properties of MGF's If <math> X_1, ...X_n</math> are iid. and <math>Y = \sum_{i=1}^n X_i. </math> then <math>M_{y}(t) = {[M_{X_1}(t)]}^n</math>. |
Revision as of 02:42, 9 January 2008
This is an activity to explore the Moment Generating Functions for the Bernoulli, Binomial, Geometric and Negative-Binomial Distributions.
- Description: You can access the applets for the above distributions at http://www.socr.ucla.edu/htmls/SOCR_DistributionFunctors.html .
- Exercise 1: Use SOCR to graph the MGF's and print the following distributions and answer the questions below. Also, comment on the shape of each one of these distributions:
- a.
- b.
- c.
- d.
- a.
Below you can see a snapshot of the MGF of the distribution of
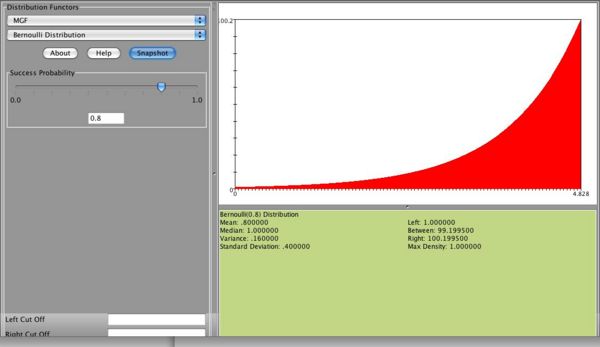
Do you notice any similarities between the graphs of these MGF's between any of these distributions?
- Exercise 2: Use SOCR to graph and print the MGF of the distribution of a geometric random variable with p = 0.2,p = 0.7. What is the shape of this function? What happens when p is large? What happens when p is small?
- Exercise 3: You learned in class about the properties of MGF's If X1,...Xn are iid. and
then
.